Annotating images at scale accurately, quickly, and cost-effectively is crucial to obtain reliable machine learning models. Thankfully, there are numerous image annotation tools that help with that. However, there are possibly hundreds of image annotation tools all purportedly offering intuitive features, fast labeling, and high accuracy. And new ones are joining the cohort every couple of months or so. This makes finding and choosing the right one difficult.
This blog will help you overcome the decision paralysis often encountered given the multiplicity of choices. We have filtered the ten best image annotation tools based on several robust factors that include ease of use, versatility, security, and quality control.
Know more: How Experts Annotate Images For AI/ML Development
Important factors to consider when choosing an image annotation tool
While most image annotation tools work similarly and have comparable capabilities, certain features and factors must be given greater consideration. Some of these include the following.
- File format support: The tool should be able to work with different image file formats such as PNG, JPG/JPEG GIF, WebP, TIFF, and BMP.
- Annotation methods: Some tools are general purpose while some are specialist and others are a compromise of the two. Each has its pros and cons. The choice should align with your needs.
- User interface: A friendly and intuitive interface can go a long way in minimizing human error as well as increasing efficiency. It should be easy to use without requiring substantial training.
- Dataset management: The tool should be able to support a high volume of data and should have functionalities like search, sort, filter, clone, and merge. Data storage is also an important factor to consider.
- Data security: Both physical and digital security need to be considered, especially if you intend to annotate personally identifiable information. Data should be accessible only to vetted annotators, and not replicable or downloadable.
- Data quality control: Built-in quality control and real-time feedback will facilitate accurate annotation. If the tool provides dashboards to track and manage issues, then that’s all the better.
- User management: A good image annotation tool must have capabilities that allow task assignment, defining supervisors, and productivity analytics.
Check: What are the artificial intelligence applications
Top 10 image annotation tools in 2024 and their pros and cons
1. CVAT
CVAT, short for Computer Vision Annotation Tool, is a free, open-source, online, interactive image and video annotation tool, initially developed by Intel. CVAT supports various image annotation types such as object detection, classification, segmentation, and LIDAR point cloud. It comes with many features like interpolation of shapes, assisted automation with deep learning, and LDAP.
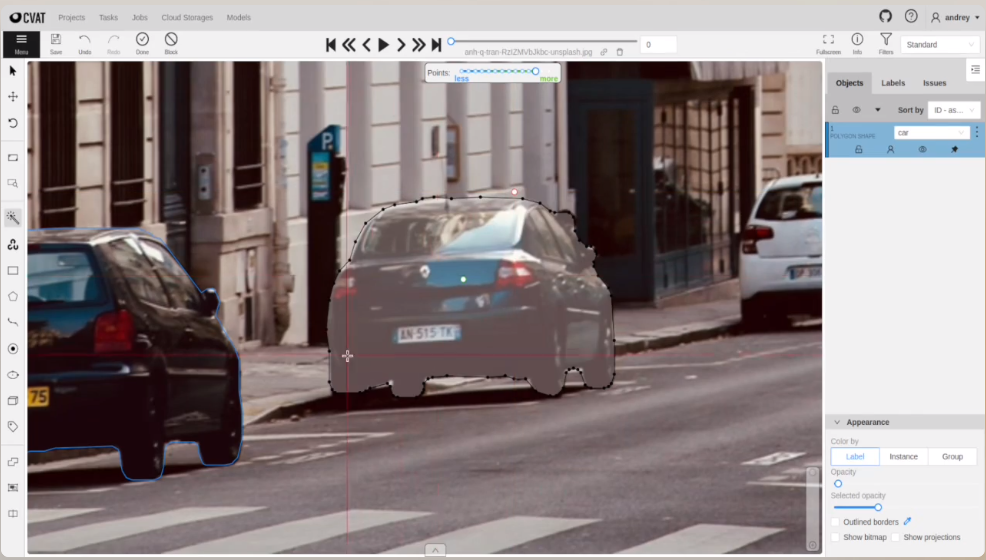
Pros:
- Intuitive user interface designed with the help of professional annotators
- Useful shortcuts for critical and frequent actions
- Equipped with analytics tools that provide useful insights
- Easy integration with AWS S3, Google Cloud Storage, and Azure Blob Storage
Cons:
- It lacks scalability and important collaborative features
- Free plan is restrictive and not suitable for large data volumes
- It doesn’t have key security features like SSO and audit trails
2. Make Sense AI
Make Sense AI is a free, open-source, online image annotation tool that lets anybody annotate images with ease. It is built on one of the most popular neural network frameworks, the TensorFlow.js engine.
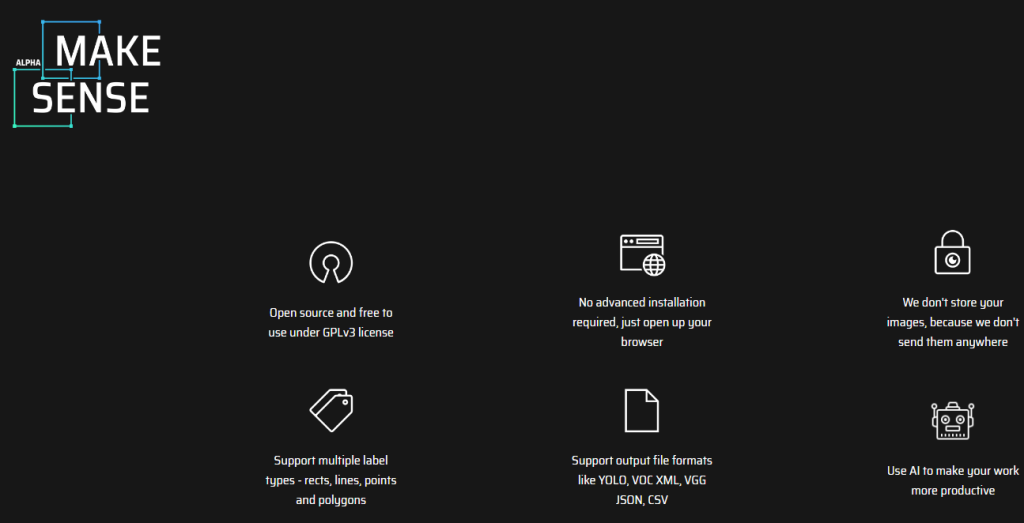
Pros:
- No installation required; can be used from a web browser
- It keeps no data about the users or the images
- It offers a cross-platform experience
- Suitable for testing and small projects
Cons:
- Functionalities are minimal
- Not ideal for large-scale annotation
- Lacks proper documentation and guide
3. SuperAnnotate
SuperAnnotate is a leading tool for pixel-perfect image annotation with advanced machine learning algorithms. It has various features and tools such as robust QA mechanisms, native integrations, data governance, and data curation. It is also a platform for building, tuning, iterating, and managing AI models.
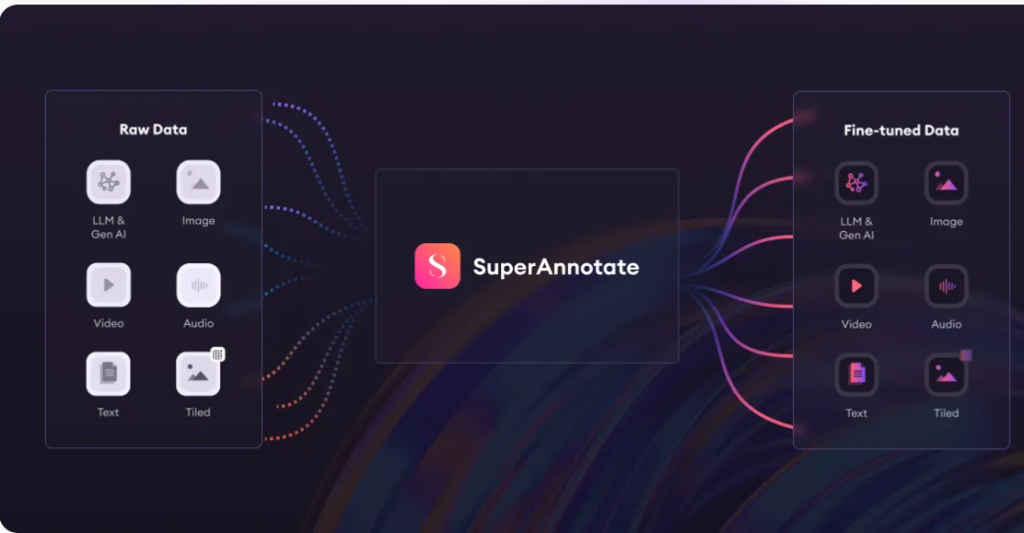
Pros:
- Highly easy to set up and use
- Excellent customer support
- Intelligent auto-labeling functions
- Allows harmonious collaboration among annotators
- Seamless integration with machine learning models
Cons:
- Cost is a barrier for those with a limited budget
- User interface not quite intuitive
- No automatic segmentation functionality
- 3D annotation capability is limited
4. Labelbox
Labelbox is a vector-based image annotation tool that supports a range of annotation types including object detection and segmentation, and offers features like zero-shot labeling and automated error analysis. It also provides features for collaboration, workforce management, reviewing annotation, and consensus labeling. The platform is used by Fortune 500 companies such as Walmart, Genentech, and Adobe.

Pros:
- Support for multiple image types and file formats
- Supports integration with data lakes
- Can be used to generate synthetic data
- Friendly and easy to use
Cons:
- Customization options are limited
- The filter system is quite limited
- Precise segmentation of labels can be challenging
- Frequent maintenance and technical support is often slow
5. Appen
Founded in 1996, it is one of the oldest companies that offers data annotation solutions. It combines cutting-edge algorithms with human intelligence to label data, which provides a reliable ground truth on which to base model training. Its clients include top technology companies such as Amazon, Apple, Facebook, and Microsoft.
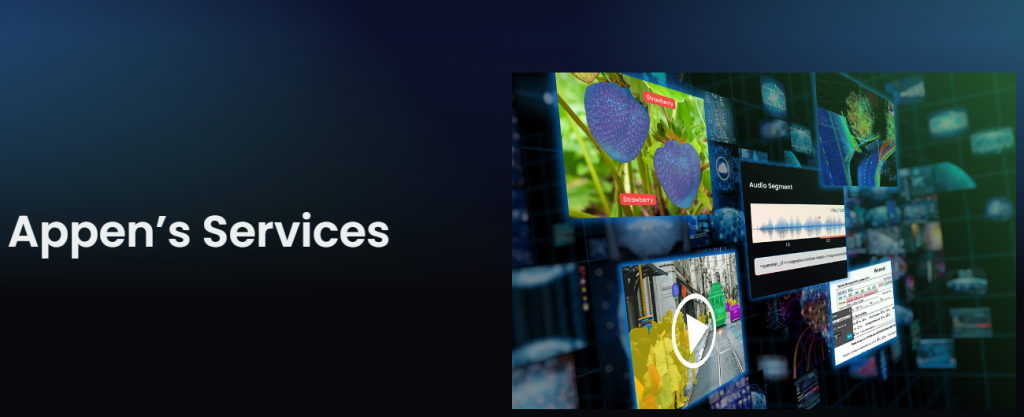
Pros:
- Multiple functionalities with support for various annotation types
- Top-notch annotation quality
- Can source raw data as well as annotate
- Good for teams looking for a complete annotation solution
Cons:
- Expensive compared to competitors
- Offers only an enterprise plan
- Limited control over the annotation process
6. V7
V7 is a user-friendly, class-agnostic annotation tool used primarily for enhanced objection detection and AI-assisted automated labeling with adaptive learning. It supports a wide range of data types but with special capability in image annotation for computer vision. It is used by enterprises like AWS, Bain & Company, Databricks, and Nvidia.
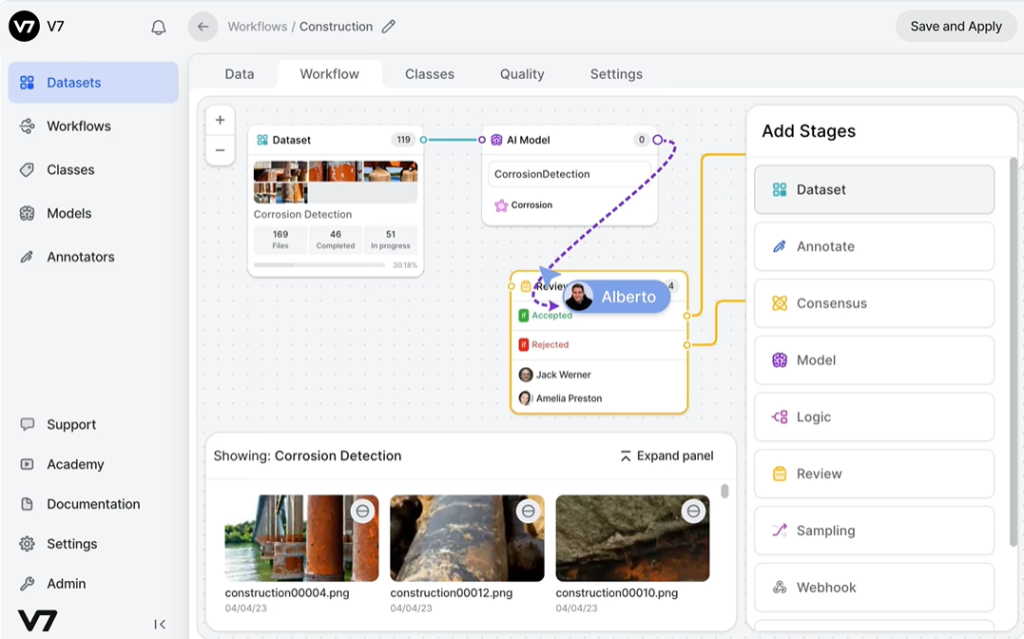
Pros:
- Ideal for projects with large datasets
- Customizable workflows
- Automated quality analysis
- Intuitive UI that’s easy to use
- Easy integration with various cloud platforms
Cons:
- Not well-optimized and therefore occasionally lags
- Export options limited to a few file formats
- Finding and filtering data is a bit of a hassle and not quite accurate
- Paid plans are expensive
7. Encord
Encord is an AI-assisted image annotation and workflow tooling platform. It is specifically designed for computer vision applications and is ideal for labeling use cases, QA workflow, and also computer vision models. It offers various annotation types including bounding box, polyline, polygon, segmentation of instances, classification, and keypoints.
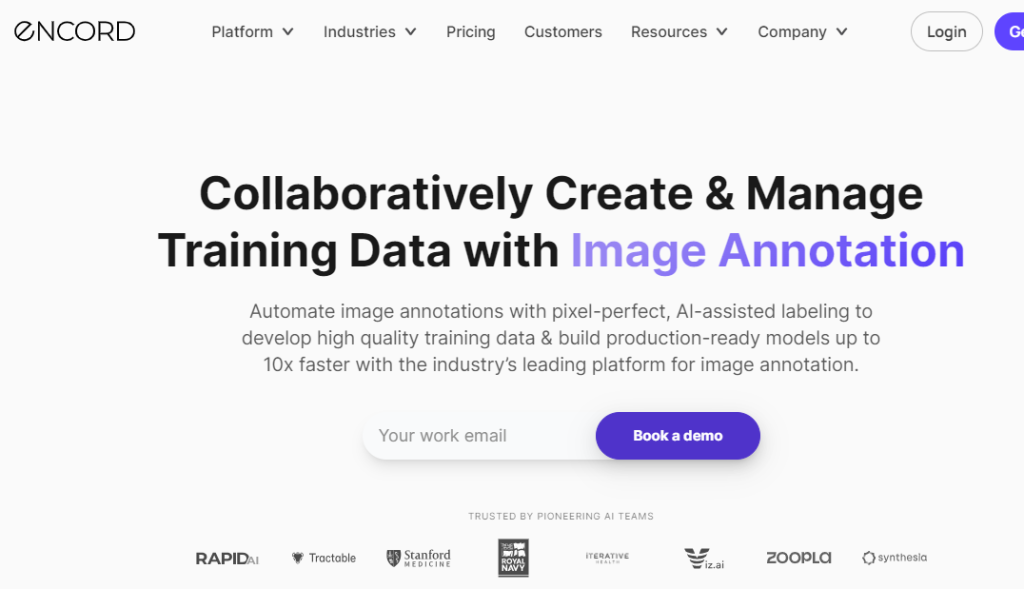
Pros:
- Customizable workflows with automated pipelines
- Provides a good balance of speed and accuracy
- Offers advanced annotation ontologies
- Equipped with in-depth performance analytics
Cons:
- Occasional lags that hinder productivity
- Documentation not quite comprehensive, which affects learning curve
- Sorting functionality not up to the mark
8. Scale
Scale, previously Scale AI, is an AI-enabled annotation tool that delivers high-quality labeling using AI with humans in the loop for quality checks. It allows annotation for a wide range of scenarios using features such as 2D and 3D bounding boxes, and semantic segmentation including in 3D. Its specialization in RLHF has made it a choice partner for leading generative AI companies such as OpenAI, Adept, and Anthropic.
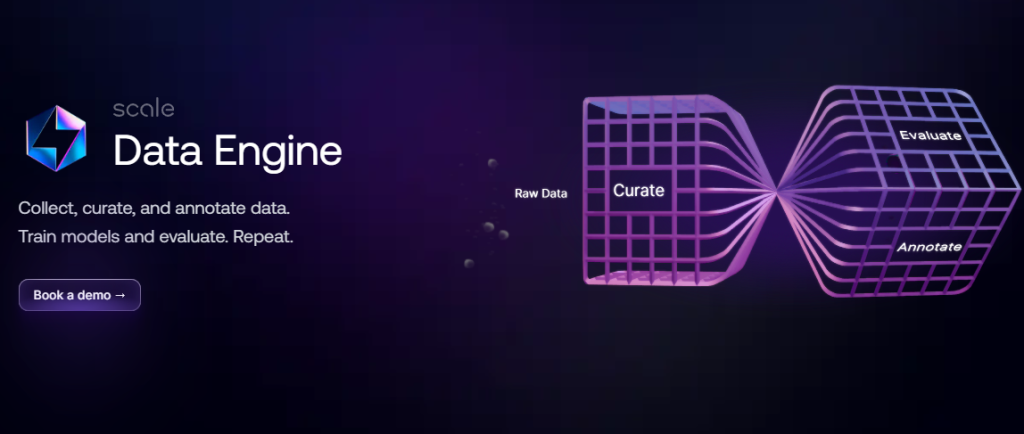
Pros:
- Robust quality control with humans in the loop
- Native integration with enterprise data as well as AWS, Azure, and Google Cloud
- Support for radar and LiDAR annotations
- Customizable workflows
Cons:
- Costlier than most other options in the market
- UI can be confusing especially for beginners
- Efficiency and accuracy fall short
9. LabelMe
LabelMe is an open-source annotation tool for images and videos created by MIT and publicly available on GitHub. It offers various annotation types including line, rectangle, circle, and polygon. It is suitable for cases that use object detection, classification, and segmentation. It is a good choice as far as open-source annotation tools go.
Pros:
- Lightweight and easy to use
Cons:
- Limited file export options
- Available only as a desktop application
- Not ideal for large projects
- Not built for collaborations
10. VGG Image Annotator (VIA)
VGG Image Annotator, or VIA, is an open-source, standalone manual annotation tool for image, video, and audio, developed by the Visual Geometry Group at the University of Oxford. It supports various annotation types like bounding boxes, polygons, keypoints, and ellipses.
Pros:
- Extremely lightweight and runs on most browsers
- Requires no setup or installation
- Simple UI that’s easy to use
- Several useful keyboard shortcuts
- Supports most file formats
Cons:
- It has no AI-assisted annotation functionality
- Handling large amounts of datasets can be cumbersome
- Collaboration and project management features are limited
- It doesn’t offer any automatic backup features
Going beyond tools
It is important to remember that the tools are mere tools. Not only do their performance depend on the humans who use them, but they are also never failsafe. And while the speed offered by automated annotation tools may be tempting, accuracy is paramount and takes precedence over speed.
A good option that provides speed with accuracy is to outsource image annotation services to a specialized image annotation company. It generally has the right tools with subject matter experts and requisite quality checks to ensure annotations are top-notch to provide the most accurate ground truth.
Conclusion
As image annotation becomes increasingly essential due to the proliferation and profusion of computer vision applications in our lives, choosing the most suitable tool to work with is an important task. While most offer similar functionalities and capabilities at the basic level, different annotation tools are designed and built with specific objectives. Choosing one whose specialty aligns with your goals would enable you to be more efficient and accurate in your image annotation tasks. When in doubt, it is a safer bet to go for a specialist than a generalist.
Frequently asked questions
Yes, several free and open-source tools exist, like VGG Image Annotator (VIA), LabelMe, LabelImg, and ImgLab. However, they have limited features and some require more technical setup compared to paid options. For small projects, however, the free ones are quite enough.
AI-enabled image annotation tools offer some benefits but they are not without drawbacks. Some of these include:
1. Potential error in annotation
2. Difficulty in annotating complex images
3. Struggle to handle edge cases
Machine learning algorithms have become highly capable at annotation and in some cases better even than human annotators. But in most cases, human supremacy remains—annotating obscure or rare cases being one. The most pragmatic approach is human-AI collaboration with semi-automatic annotation. This offers speed without sacrificing accuracy.
Author Bio:
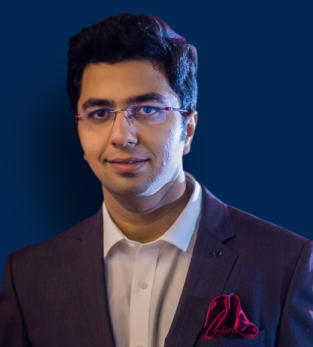
Rohit Bhateja is the Director of the Data & Digital division at SunTec India. He has a decade-long career in management and growth planning with core expertise in digital marketing, customer acquisition, marketing analytics, and brand communication. He likes exploring data trends to devise transformative marketing solutions. At SunTec India, he has successfully led teams, redesigned business processes, and solved complex business challenges.
Rohit likes keeping tabs on the latest data trends and solutions that are shaping the industry & transforming business. He is also an avid writer with a penchant for translating his insights and understanding about any matter at hand into articles that promote a growth-driven mindset.